Due to their complexity, many processes cannot be automated, or not fully automated, using conventional technical solutions, because the operational control of these processes requires the know-how (often accumulated over years) of experts. This is also the case when raising shrimp. Determining the number and size of the shrimp is crucial for good system control, e.g. B. for the feed supply, the yield and the economic performance of the farm. If e.g. For example, if too much feed is added, the water quality deteriorates, if too little feed is added, the growth of the animals is inhibited. In order to determine the biomass, manual water samples are taken regularly and estimates and forecasts are made based on these samples. In order to optimize control and minimize manual effort, we have implemented continuous data acquisition and evaluation using methods from computer vision, machine learning and the Internet of Things.
Combination of computer vision methods with the Internet of Things
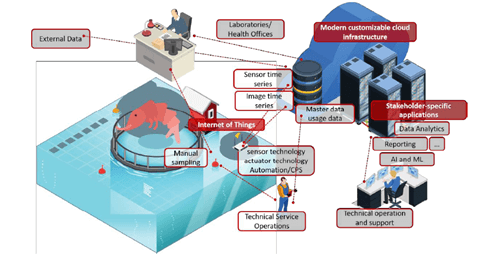
The procedure can be divided into three main topics:
1. Preprocessing (local, or "on the edge")
A local computer uses an underwater camera to record and filter data in a tank. A shrimp detection is carried out, which is implemented using machine learning methods. If a shrimp is detected in the recording area, this sequence will be saved as a video file and uploaded to the cloud.
2. Analysis of the pre-processed data (global, or "in the cloud")
The filtered data is then further processed in the cloud. The image data are z. B. used to estimate the size of individual shrimp, which makes it possible to track and document the growth process.
3. Adjustment of the control system
Based on the generated and already existing data, the control system of the shrimp farm is optimized. All existing data from laboratory results to technical specifications are integrated.
To use
The designed concept combines computer vision with concepts of the Internet of Things. This combination makes it possible to generate new input parameters for control systems in various application areas. A possible loss of information and the use of unsystematic reference values are reduced and thus enable the automation of these processes. This means that the manual effort for control can be significantly or even completely reduced by in-situ measurements. At the same time, it is possible to compare data, control parameters and the performance of different locations, to identify best practices and to exchange them across locations.
This project was carried out as part of the European project Digital Innovation Hubs for Cyber-Physical Systems (DIH4CPS). The grant number is 872548.